Example Regression
Written on July 20th, 2021 by control lab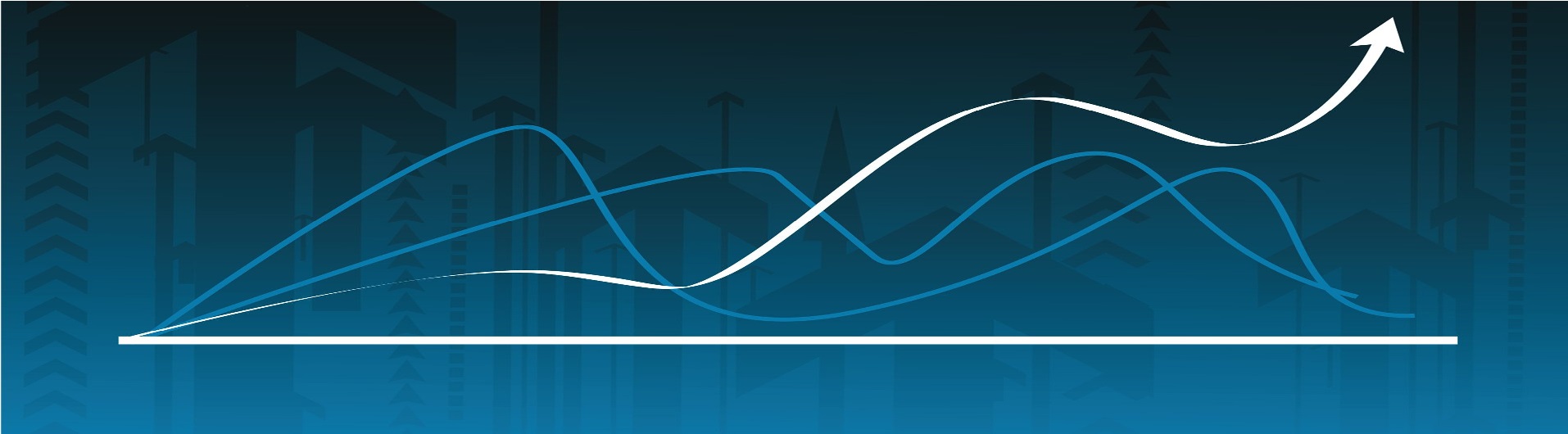
This example shows a comparative analysis for various simple kernels, such as RBF, Poly, and linear. We generated the data and showed how the decision function depends on the kernel. TKL proved to be quite effective.
import numpy as np
import matplotlib.pyplot as plt
from sklearn import svm, datasets
from PMKLpy import PMKL
num = 100
x = 10.*np.random.rand(num,1)
y = x + np.sin(x)+5*np.sign(x-5)+ np.random.rand(num,1)-0.5
C = 1.0
eps = 0.1
SVM = PMKL.PMKL( C=C, epsilon= eps, to_print = False)
models = [svm.SVR(kernel='linear', C=C, epsilon = eps),
svm.SVR(kernel='rbf', gamma=0.7, C=C, epsilon = eps),
svm.SVR(kernel='poly', degree=3, gamma='auto', C=C, epsilon = eps),
SVM]
models = [clf.fit(x, y) for clf in models]
titles = ['SVR with linear kernel',
'SVR with RBF kernel',
'SVR with polynomial (degree 3) kernel',
'SVR with TKL kernel']
fig, sub = plt.subplots(1, 4, figsize = (25, 5))
plt.subplots_adjust(wspace=0.4, hspace=0.4)
xx = np.arange(0, 10, 0.1)[:, np.newaxis]
for clf, title, ax in zip(models, titles, sub.flatten()):
yy = clf.predict(xx)
ax.plot(xx, yy, color = 'r', label = 'Desicion function')
ax.scatter(x, y, c= 'b', marker = '+', label = 'Data Points')
ax.set_xlim(xx.min(), xx.max())
ax.set_ylim(yy.min(), yy.max())
ax.set_xlabel('x')
ax.set_ylabel('y')
ax.legend()
ax.set_title(title)
Feel free to share!